المجلة الدولية للعلوم والتقنية
International Science and Technology Journal
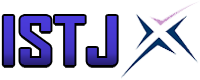
ISSN: 2519-9854 (Online)
ISSN: 2519-9846 (Print)
مجلة علمية محكّمة تهتم بنشر البحوث والدراسات في مجال العلوم التطبيقية، تصدر دورياً تحت إشراف نخبة من الأساتذة
الكشف التلقائي عن مخالفات البناء باستخدام صور الطائرات بدون طيار وتقنيات الذكاء الاصطناعي: دراسة تطبيقية في مدينة جدة
الباحث(ون): | - أ. محمد يوسف المنتشري
- أ. د. مفرح بن ضايم القرادي
- د. عادل سعيد الشمراني
|
المؤسسة: | قسم الجغرافيا ـ جامعة الملك سعود (المملكة العربية السعودية) قسم الجغرافيا ـ جامعة الملك سعود (المملكة العربية السعودية) كلية علوم وهندسة الحاسب ـ جامعة جدة (المملكة العربية السعودية) |
المجال: | الاستشعار عن بعد و الجيولوجيا والجيوفيزيائية وعلوم الارض |
منشور في: | العدد الثالث و الثلاثون - أكتوبر 2023 |
الملخص
تهدف هذه الدراسة إلى تدريب واختبار نموذج تعلم عميق باستخدام تقنيات الذكاء الاصطناعي you only look once version 4 (YOLOv4)من أجل الكشف التلقائي عن مخالفات بناء الملاحق من صور الطائرة بدون طيار في الوقت الفعلي في حي الأجاويد بمدينة جدة. تم تدريب النموذج على مجموعة بيانات تدريبية تحتوي على 800 صورة وتم استخدام موارد الحوسبة السحابية من Google Colab. بعد التدريب، تم تطبيق النموذج على صور الدرون لاكتشاف المخالفات في منطقة الدراسة. حيث تم رصد 256 عينة، منها 67 مبنى مخالف حيث تتجاوز فيها مساحة بناء الملحق عن 50% مقابل 189 مبنى لم يكن فيه مخالفة. أظهر النموذج دقة عالية في التنبؤ بالمخالفات بنسبة 91٪ و87٪، وكذلك دقة استدعاء عالية بنسبة 98٪ و93٪وبدرجة F1 بنسبة 0.95 و 0.90 وقدرة على تحديد التداخل المكاني بنسبة 84٪ و79٪ في مجموعتي بيانات التدريب والاختبار على التوالي. كما أظهر النموذج دقة هندسية متوسطة بنسبة 7.1٪. خلصت الدراسة إلى أهمية التقنيات الحديثة في دعم مراقبة مخالفات البناء، وتشير إلى إمكانية استخدام نموذج YOLOv4 المدرب لاكتشاف المخالفات وانتهاكات اشتراطات البناء بالوقت الفعلي.
الكلمات المفتاحية: الكشف التلقائي، مخالفات البناء، الوقت الفعلي، صور الطائرات بدون طيار الذكاء الاصطناعي، YoloV4، مدينة جدة.
Abstract
This study aims to train and test a deep learning model using artificial intelligence techniques, specifically You Only Look Once version 4 (YOLOv4), for real-time automatic detection of building extension violations from Drone images in Al-Ajaweed district )Jeddah city(. The model was trained on a dataset of 800 images, and cloud computing resources from Google Colab were utilized. After training, the model was applied to Drone images to detect violations in the study area, where 256 samples were monitored. Among these samples, 67 buildings had violations, with the extension area exceeding 50%, while 189 buildings had no violations.The model showed high accuracy in predicting violations at 91% and 87%, as well as high recall accuracy at 98% and 93%, with an F1 score of 0.95 and 0.90. It also demonstrated the ability to detect spatial overlap at 84% and 79% in the training and test datasets, respectively. Furthermore, it showed an average geometric accuracy of 7.1%.The study emphasized the significance of modern technologies in supporting building violation monitoring and highlighted the potential of the trained YOLOv4 model for real-time detection of violations and non-compliance with building regulations.
Keywords: Automatic detection, building violations, real-time, aerial Drone images, artificial intelligence, YOLOv4, Jeddah city.