مجلة مفتوحة الوصول
المجلة الدولية للعلوم والتقنية
International Science and Technology Journal
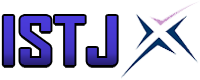
ISSN: 2519-9854 (Online)
ISSN: 2519-9846 (Print)
مجلة علمية محكّمة تهتم بنشر البحوث والدراسات في مجال العلوم التطبيقية، تصدر دورياً تحت إشراف نخبة من الأساتذة
ينشرها
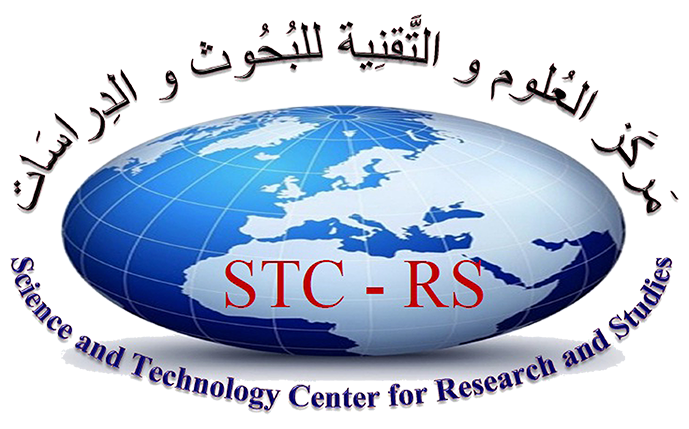
تحت إشراف
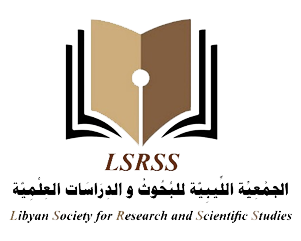
Challenges and Opportunities in Federated Learning
الباحث(ون): | - Ahmed S Alardawi
- Ammar Odeh
- Abobakr Aboshgifa
- Nabil Belhaj3
|
المؤسسة: | The College of Computer Technology, Tripoli-Libya Department of Computer Science Princess Sumaya University for Technology, Amman, Jordan The Libyan higher technical center for training and production, Tripoli, Libya |
المجال: | علوم الحاسوب و النظم الخبيرة وتقنية المعلومات |
منشور في: | العدد الخامس و الثلاثون - أكتوبر 2024 |
الملخص
التعلم الفدرالي (FL) هو إطار تعلم آلي يتيح تدريب النماذج بشكل تعاوني عبر عدة أجهزة حافة أو خوادم لامركزية، مع الحفاظ على البيانات محلية وخاصة. هذه الطريقة تلغي الحاجة إلى تبادل البيانات الحساسة، مما يعزز الخصوصية والأمان. يستفيد التعلم الفدرالي من الطبيعة الموزعة لتوليد البيانات من أجل تحسين فعالية التعلم. يتناول هذا البحث المفاهيم الأساسية للتعلم الفدرالي، مع التركيز على كيفية تمكينه للتعلم الجماعي دون مركزية البيانات الفردية. وهذا أمر بالغ الأهمية في المجالات التي تتطلب خصوصية البيانات، مثل الرعاية الصحية والخدمات المالية. كما يناقش البحث الآليات التقنية للتعلم الفدرالي، بما في ذلك الخوارزميات المستخدمة في التدريب اللامركزي، وتقنيات تجميع البيانات، ودور الخادم المركزي عند الحاجة. في التعلم الفدرالي، تقوم الأجهزة بحسابات على البيانات المحلية وتشارك فقط التحديثات أو التدرجات الخاصة بالنموذج، مما يقلل من خطر تعريض المعلومات الحساسة. للتعلم الفدرالي تطبيقات متنوعة، مثل تمكين نماذج تشخيص الأمراض في الرعاية الصحية دون الحاجة إلى مشاركة بيانات المرضى، وتعزيز الخصوصية في تخصيص ميزات الأجهزة المحمولة. ومع ذلك، يواجه التعلم الفدرالي تحديات، بما في ذلك قضايا مزامنة التحديثات، والتعامل مع البيانات غير المستقلة والمتجانسة عبر الأجهزة، وضمان مقاومة الهجمات العدوانية. يتناول البحث هذه التحديات ويفحص الاستراتيجيات لتجاوزها، مقدماً نظرة شاملة على إمكانات التعلم الفدرالي وتطبيقاته المستقبلية [1، 2] ........................
الكلمات المفتاحية:........... التعلم الفيدرالي، الخصوصية، الأمان، اللامركزي، التعلم الآلي، الأجهزة الطرفية
Abstract
Federated Learning (FL) is a machine learning framework that allows collaborative model training across multiple decentralized edge devices or servers while keeping the data local and private. This method eliminates the need to exchange sensitive data, enhancing privacy and security. FL leverages the distributed nature of data generation for more effective learning. The paper explores the foundational concepts of FL, focusing on how it enables collective learning without centralizing individual data. This is particularly important in sectors where data privacy is critical, such as healthcare and finance. It discusses the technical mechanisms of FL, including the algorithms for decentralized training, data aggregation techniques, and the role of a central server, when applicable. In FL, devices compute on local data and share only model updates or gradients, reducing the risk of exposing sensitive information. FL has diverse applications, such as enabling disease diagnosis models in healthcare without sharing patient data and enhancing privacy in mobile device personalization. However, FL also faces challenges, including issues with synchronization of updates, handling non-IID (independent and identically distributed) data across devices, and ensuring resistance to adversarial attacks. The paper addresses these challenges and examines strategies to overcome them, providing a comprehensive overview of FL's potential and its future applications [1, 2]................
Keywords: ...............Federated Learning, Privacy, Security, Decentralized, Machine Learning, Edge Devices