Open Access Journal
International Science and Technology Journal
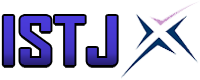
ISSN: 2519-9854 (Online)
ISSN: 2519-9846 (Print)
A peer-reviewed and open access journal concerned with publishing researches and studies in the field of applied sciences and engineering
Published by
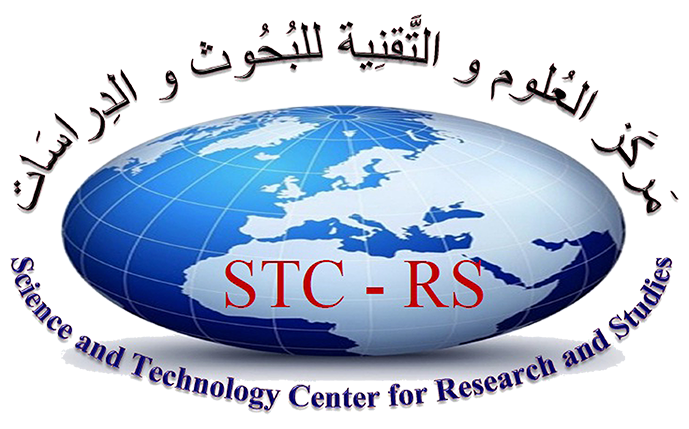
Supervised by
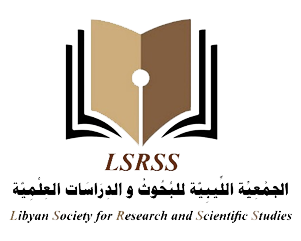
Distance Based Clustering for K-mean Algorithm
Researcher(s): | - Ahmed Elajeli Rgibi
- Ali Mokhtar Shafah
|
Institution: | Faculty of Engineering-Sabratha University Faculty of Economic Zawiya University2 |
Field: | علوم الحاسوب و النظم الخبيرة وتقنية المعلومات |
Published in: | 25th volume - April 2021 |
الملخص
تعتبر تقنيات تصنيف البيانات الى مجموعات متشابهة مهمة جداً لتصنيف البيانات الغير معنونة. حيث يتم تجميع البيانات او الكائنات الى مجموعات مختلفة، كل مجموعة تحتوي على البيانات او الكائنات المتشابهة. من أهم هذه التقنيات خوارزمية K-mean, حيث تعتبر من أكثر خوارزميات التصنيف شيوعاً واستخداماً وايضا تتميز بسهولة الاستخدام. الا ان هذه الخوارزمية تحتوي على بعض العيوب، من أهم هذه العيوب هو اختيار النقاط الاولية بطريقة عشوائية. ان الاختيار العشوائي يؤثر سلباً على اداء الخوارزمية وكذلك عدد مرات التكرار للوصول الى الحل الامثل. في هذه الورقة قدمنا اقتراح يتم من خلاله ايجاد قيم النقاط الابتدائية وذلك باستخدام النقاط المتباعدة كنقاط اولية، يتم تطبيق هذا المقترح من خلال خوارزمية النقاط المتباعدة التي صممت من اجل تحقيق الهدف من هذه الورقة، وباستخدام هذه الطريقة قد تم تحقيق نتائج أفضل مقارنة مع الطريقة التقليدية التي تستخدم الاختيار العشوائي.
Abstract
Clustering is considered as a significant technique of classifying various groups of datasets. In this technique, the similar data objects are grouped together relocated in a new cluster .For this purpose, The K-means algorithm Cluster analysis is used .Yet, it still has several disadvantages: First, attaining the appropriate solutions. Second, results significantly affected by the selection of initial centroid points .Third, number of clusters has to be identified in advance. So the initial centroid for clustering is a key factor in k-Means. In this paper, a selection of points as initial centroids based on the distance is used instead of the random selection procedure. Precisely, the furthest apart points were selected for such a purpose .This proposed method has achieved better clustering process .Consequently, the number of repetitions is decreased, and the optimal solution is achieved