Open Access Journal
International Science and Technology Journal
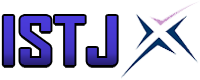
ISSN: 2519-9854 (Online)
ISSN: 2519-9846 (Print)
A peer-reviewed and open access journal concerned with publishing researches and studies in the field of applied sciences and engineering
Published by
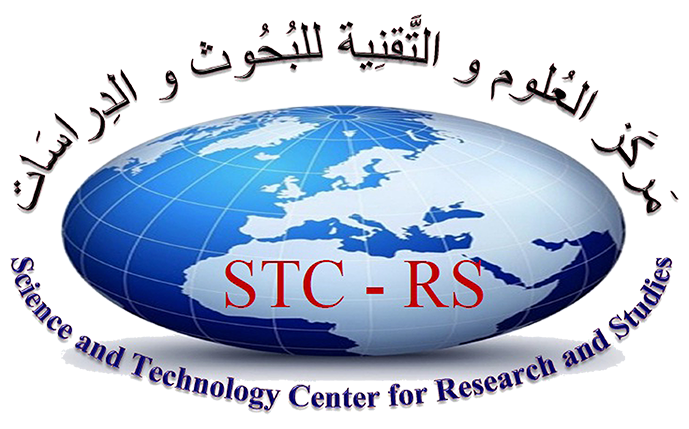
Supervised by
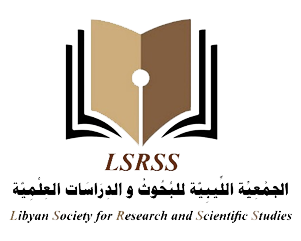
Early diagnosis system to detect phosphorus deficiency in barley fields Based on an Improved YOLO-v8 Network
Researcher(s): | - Ababaker Noureldeen
- Mohsen Nouralddeen
- Elhabeb Abubker
- Mohamed Abubker
- Abdarahmah Aboseta
|
Institution: | Higher Institute of science and Technology Tamzawa Ashati, Libya Faculty of Information Technology Wadi Al-Shati University Libya College of Technical Sciences Alshati, Libya College of Sciences and Technology, ALzweah ,Libya |
Field: | علوم الحاسوب و النظم الخبيرة وتقنية المعلومات |
Published in: | 34th volume - April 2024 |
الملخص
تقدم هذه الدراسة طريقة تشخيصية للكشف المبكر والسريع عن نقص الفسفور (P) في حقول الشعير في البيئة الطبيعية، وذلك من خلال الرؤية الحاسوبية وعلى أساس نهج التعلم العميق. ويتم ذلك عن طريق إنشاء نموذج تدريب يعتمد على شبكة YOLOv8. يتم استخدام طريقة التنميط الظاهري للتعرف على النبات، ومن ثم اعتماداً على لون الأوراق والسيقان للكشف عن نقص الفوسفور.(P) تم إجراء بعض التحسينات على الخوارزمية الأساسية، باستخدام تقنيات معالجة الصور مثل التقليب والتدوير والالتفاف العميق وتغيير الحجم لإثراء الميزات. في نهجنا، تم تصنيف الصور باستخدام مربعات محيطة متعددة الأضلاع بالتعاون مع خبراء زراعيين لتحديد مجالات الاهتمام للنموذج. تم تقسيم مجموعة البيانات إلى التحقق والتدريب والاختبار. وفي المرحلة التجريبية، قمنا بتحليل أداء النموذج باستخدام مجموعة من مقاطع الفيديو الملتقطة بكاميرا الهاتف المحمول كمرحلة أولى، وحقق نموذجنا دقة كشف تصل إلى 80%. وفي المرحلة الثانية قمنا باختبار النموذج باستخدام الصور ومقاطع الفيديو المأخوذة من مجموعة كاميرات مثبتة أعلى آلة الري المحوري للاستفادة من حركة الآلة في مسح الحقل بالكامل. حقق النموذج دقة كشف تصل إلى 65%. ولذلك، فإن الطريقة المقترحة يمكن أن توفر نظام التنبؤ المبكر لاحتياجات النبات، مما يساعد المزارعين على الحفاظ على صحة المحاصيل واختيار الأسمدة المناسبة.
الكلمات المفتاحية: الفوسفور (P)، التعلم العميق، YOLOv8، الرؤية الحاسوبية، معالجة الصور.
Abstract
This study presents a diagnostic method for early and rapid detection of phosphorus (P) deficiency in barley fields in the natural environment, stemming from computer vision and based on a deep learning approach. This is done by creating a training model based on the YOLOv8 network. A phenotyping method is used to identify the plant, and then based on the color of the leaves and stems to detect phosphorus (P) deficiency. Some improvements have been made to the basic algorithm, using image processing techniques such as flipping, rotating, deep convolution, and resizing for feature enrichment. In our approach, images were classified using polygonal bounding boxes in collaboration with agricultural experts to identify areas of interest for the model. The dataset was divided into validation, training, and testing. In the experimental phase, we analyzed the model's performance using a set of video clips captured with a mobile phone camera as a first stage, and our model achieved a detection accuracy of up to 80%. In the second stage, we tested the model using images and video clips taken from a group of cameras installed on top of the pivot irrigation machine to take advantage of the machine’s movement to scan the entire field. The model achieved a detection accuracy of up to 65%. Therefore, the proposed method can provide an early prediction system for plant needs, helping farmers maintain crop health and choose appropriate fertilizers.
Keywords: Phosphorus (P), deep learning, YOLOv8, computer vision, image processing.